Automatic Analysis of Large-scale Nanopore Data Using Hidden Markov Models
Peer reviewed, Journal article
Published version
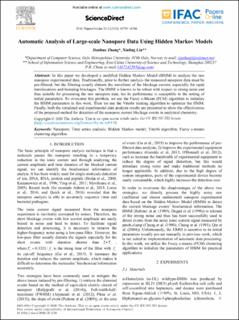
Permanent lenke
https://hdl.handle.net/11250/2993686Utgivelsesdato
2020-04-14Metadata
Vis full innførselSamlinger
Originalversjon
IFAC-PapersOnLine. 2020, 53 (2), 16759-16766. https://doi.org/10.1016/j.ifacol.2020.12.1138Sammendrag
In this paper we developed a modified Hidden Markov Model (HMM) to analyze the raw nanopore experimental data. Traditionally, prior to further analysis the measured nanopore data must be pre-filtered, but the filtering usually distorts the waveform of the blockage current, especially for rapid translocations and bumping blockages. The HMM is known to be robust with respect to strong noise and thus suitable for processing the raw nanopore data, but its performance is susceptible to the setting of initial parameters. To overcome this problem, we use the Fuzzy c-Means (FCM) algorithm to initialize the HMM parameters in this work. Then we use the Viterbi training algorithm to optimize the HMM. Finally, both the simulated and experimental data analysis results are presented to show the effectiveness of the proposed method for detection of the nanopore current blockage events in analytical chemistry.